Training Module on the Evaluation of Best Modeling Practices
PDF version of this training | All modeling training modules
Best Modeling Practices: Evaluation
This module builds upon the fundamental concepts outlined in previous modules: Environmental Modeling 101 and The Model Life-cycle. The objectives of this module are to explore the topic of model evaluation and identify the 'best modeling practices' and strategies for the Evaluation Stage of the modelmodelA simplification of reality that is constructed to gain insights into select attributes of a physical, biological, economic, or social system. A formal representation of the behavior of system processes, often in mathematical or statistical terms. The basis can also be physical or conceptual. life-cycle.
Model Evaluation
According to the EPA (2009a) model evaluation is defined as:
"The process used to generate information that will determine whether a model and its analytical results are of a sufficient quality to inform a decision."
The process of evaluation is used to address the:
- soundness of the underlying science of the model
- quality and quantity of available data
- degree of correspondence between model output and observed conditions
- appropriateness of a model for a given application
Best Modeling Practices for Model Evaluation:
All models (especially regulatory models) should be continually evaluated at all stages within their life-cycle. The Guidance on the Development, Evaluation, and Application of Environmental Models (EPA, 2009a) describes best practices for model evaluation that include the following activities:
- Quality Assurance (QA) project planning
- Peer review
- Model corroboration
- Sensitivity analysis
Sensitivity analysisThe computation of the effect of changes in input values or assumptions (including boundaries and model functional form) on the outputs. The study of how uncertainty in a model output can be systematically apportioned to different sources of uncertainty in the model input. By investigating the "relative sensitivity" of model parameters, a user can become knowledgeable of the relative importance of parameters in the model. (SA)
- Uncertainty analysis
Uncertainty analysisInvestigates the effects of lack of knowledge or potential errors on the model (e.g., the "uncertainty" associated with parameter values) and when conducted in combination with sensitivity analysis (see definition) allows a model user to be more informed about the confidence that can be placed in model results. (UA)
Justification
Why Are Models Evaluated?
There is inherent uncertaintyuncertaintyThe term used in this guidance to describe lack of knowledge about models, parameters, constants, data, and beliefs. There are many sources of uncertainty, including the science underlying a model, uncertainty in model parameters and input data, observation error, and code uncertainty. Additional study and collecting more information allows error that stems from uncertainty to be minimized/reduced (or eliminated). In contrast, variability (see definition) is irreducible but can be better characterized or represented with further study. associated with models which can lead to (Sunderland, 2008):
- Extreme and inefficient approaches to problem solving
- Delays in decision making which require model support
- Unintended consequences from misinformed decisions
Model evaluation provides the model development team (developers, intended users, and decision makers) with the level of model corroboration; providing an understanding of how consistent the model is with data.
Model corroboration is defined as the quantitative and qualitative methods for evaluating the degree to which a model corresponds to reality (e.g. measured data). In general, the term corroboration is preferred (rather than validationvalidationConfirmation by examination and provision of objective evidence that the particular requirements for a specific intended use are fulfilled. In design and development, validation concerns the process of examining a product or result to determine conformance to user needs. OEI: An analyte- and sample-specific process that extends the evaluation of data beyond method, procedural, or contractual compliance (i.e., data verification) to determine the quality of a specific data set.) because it implies a claim of usefulness and not truth (EPA, 2009a).
Model evaluation can provide answers to questions like:
- Does the model reasonably approximate the system?
- Is the model supported by the available data?
- Is the model founded with principles of sound science?
- Does the model perform the specified task?
- What level of uncertainty is attributable to the data or the model?
- Is better data needed for future model applications?
Evaluation Techniques
There are many techniques and approaches for model evaluation, as made evident by a review conducted by researchers in the EPA Office of Research and Development. Their efforts (Matott et al., 2009) identified 70 model evaluation tools, classified into seven thematic categories:
- Data Analysis: to evaluate or summarize input, response or model output data
- Identifiability Analysis: to expose inadequacies in the data or suggest improvements in the model structure
- Parameter Estimation: to quantify uncertain model parameters
parametersTerms in the model that are fixed during a model run or simulation but can be changed in different runs as a method for conducting sensitivity analysis or to achieve calibration goals. using model simulations and available response data
- Sensitivity Analysis: to determine which inputs are most significant
- Uncertainty Analysis: to quantify output uncertainty by propagating sources of uncertainty through the model
- Multi-model Analysis: to evaluate model uncertainty or generate ensemble predictions via consideration of multiple plausible models
- Bayesian Networks: to combine prior distributions of uncertainty with general knowledge and site-specific data to yield an updated (posterior) set of distributions
Further Insight:
Evaluating uncertainty in integrated environmental models: A review of concepts and tools. Matott, L. S., J. E. Babendreier and S. T. Purucker 2009. Water Resour. Res. 45: Article Number: W06421.
Best Practice: Graded Approach
Model evaluation should be conducted using a graded approach that is adequate and appropriate to the model application or decision at hand (EPA, 2009a).
A graded approach recognizes that model evaluation, as an iterative process, cannot be designed in a 'one size fits all approach.' Further, the NRC (2007) recommends that model evaluation be designed to the complexity and impacts (of the model) in addition to consideration of the life-cycle stage of the model and the evaluation history.
For example, a screening model (a type of model designed to provide a "conservative" or risk-averse answer) that is used for risk management should undergo rigorous evaluation to avoid false negatives, while still not imposing unreasonable data-generation burdens (false positives) on the regulated community.
Ideally, decision makers and modeling staff work together at the onset of new projects to identify the appropriate degree of model evaluation.
Intended Use of Model Results

- Regulatory compliance
- Litigation
- Congressional testimony
- Regulatory development
- State Implementation plan attainment
- Verification of model
- Trends monitoring (non-regulatory)
- Proof of principle
- Basic research
- Bench-scale testing
A Graded Approach to Model Evaluation. Model results that have higher consequences our outcomes should be subjected to more rigorous evaluation methods.
A Model Evaluation Plan
An evaluation plan can be a valuable tool for outlining the evaluation exercises that will be carried out during the model life-cycle. The evaluation plan should be determined during the earliest stages of model development (i.e. in the required QA project plan) and include all members of the model development team.
Model Development Team:
Comprised of model developers, users (those who generate results and those who use the results), and decision makers; also referred to as the project team.
Recommendations of Elements to Include in an Evaluation Plan (NRC, 2007)
- Describe the model and its intended uses
- Describe the relationship of the model to data (for both inputs and corroboration)
- Describe how model performance will be assessed
- Use an outline or diagram that show how the elements and instances of evaluation relate to the model's life cycle
- Describe the factors or events that might trigger the need for major model revisions or the circumstances that might prompt users to seek an alternative model. These can be fairly broad and qualitative.
- Identify the responsibilities, accountabilities, and resources needed to ensure implementation of the evaluation plan.
A Model Evaluation Plan (cont.)
The over arching goal of model evaluation is to ensure model quality. The EPA defines quality in the Information Quality Guidelines (IQGs) (EPA, 2002b). The IQGs apply to all information that EPA disseminates, including models, information from models, and input data.
Additional Web Resource:
Further guidance on model evaluation can be found in another module: Sensitivity and Uncertainty Analyses.
Information Quality Guidelines
Quality has three major components: integrityintegrityOne of three main components of quality in EPA's Information Quality Guidelines. Integrity refers to the protection of information from unauthorized access or revision to ensure that the information is not compromised through corruption or falsification., utility
utilityOne of three main components of quality in EPA's Information Quality Guidelines. Utility refers to the usefulness of the information to the intended users., and objectivity
objectivityOne of three main components of quality in EPA's Information Quality Guidelines. Objectivity includes whether disseminated information is being presented in an accurate, clear, complete and unbiased manner. In addition, objectivity involves a focus on ascertaining accurate, reliable and unbiased information. (EPA, 2002b). In the context of environmental modeling, evaluation aims to ensure the objectivity of information from models by considering their accuracy
accuracyCloseness of a measured or computed value to its "true" value, where the "true" value is obtained with perfect information. Due to the natural heterogeneity and stochasticity of many environmental systems, this "true" value exists as a distribution rather than a discrete value. In these cases, the "true" value will be a function of spatial and temporal aggregation., bias
biasSystematic deviation between a measured (i.e., observed) or computed value and its "true" value. Bias is affected by faulty instrument calibration and other measurement errors, systematic errors during data collection, and sampling errors such as incomplete spatial randomization during the design of sampling programs., and reliability
reliabilityThe confidence that (potential) users have in a model and in the information derived from the model such that they are willing to use the model and the derived information. Specifically, reliability is a function of the performance record of a model and its conformance to best available, practicable science..
Case Study
The Atmospheric Modeling and Analysis Division
(AMAD Website)
The Atmospheric Modeling and Analysis Division (AMAD) of the Office of Research and Development leads the development and evaluation of predictive atmospheric models on all spatial and temporal scales for assessing changes in air quality and air pollutant exposures. For evaluation of their models, AMAD has developed a framework to describe different aspects of model evaluation:
Framework to describe different aspects of model evaluation:
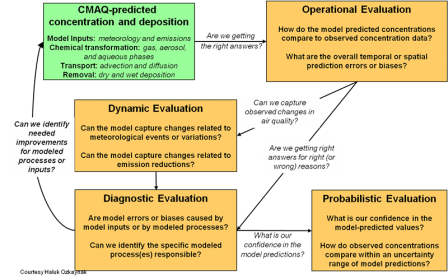
A model evaluation framework example for the CMAQ model. Adapted from the AMAD Website. (Click on image for a larger version)
Case Study (cont.)
- Operational evaluation: a comparison of model-predicted and routinely measured concentrations of the end-point pollutant(s) of interest in an overall sense. (See example in right page.)
- Diagnostic evaluation: investigates the atmospheric processes and input drivers that affect model performance to guide model development and improvements needed in emissions and meteorological data.
- Dynamic evaluation: assesses a model's air quality response to changes in meteorology or emissions, which is a principal use of an air quality model for air quality management.
- Probabilistic evaluation: characterizes uncertainty of model predictions for model applications such as predicted concentration changes in response to emission reductions.
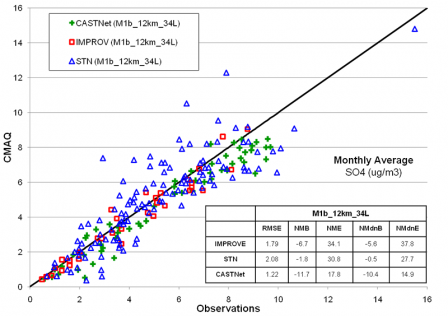
An example of operational evaluation - a fundamental first phase of any model evaluation. A scatter plot of observed versus CMAQ (an air quality model) predicted sulfate (SO4) concentrations for August 2006. (Image modified from AMAD) (Click on image for a larger version)
QA Planning
Quality Assurance Planning
A well-executed quality assurance project plan (QAPP) helps to ensure how model evaluation will be performed and that a model performs the specified task. The objectives and specifications of the model set forth in a quality assurance plan can also be subjected to peer review.
Data quality assessments are an integral component of any QA plan that includes modeling activities. Similar to peer review, data quality assessments evaluate and assure that (EPA, 2002a):
- the data used by model is of high quality
- data uncertainty is minimized
- the model has a foundation of sound scientific principles
The data used to parameterize and corroborate models should be assessed during all relevant stages of a modeling project. These data assessments should be both qualitative and quantitative (i.e. is there enough appropriate data). These assessments ensure that the data sufficiently represent the system being modeled.
Additional Web Resources
The topic of model documentation (an important component of a complete QA plan) is discussed in other modules as well:
Additional information (including guidance documents) can be found at the Agency's website for the Quality System for Environmental Data and Technology.
Drivers for QA Planning
Congress has directed the Office of Management and Budget (OMB) to issue government-wide guidelines that
"...provide policy and procedural guidance to Federal agencies for ensuring and maximizing the quality, objectivity, utility, and integrity of information (including statistical information) disseminated by Federal agencies..."
EPA is dedicated to the collection, generation, and dissemination of high quality information (EPA, 2002b). The EPA states that its quality systems must include:
"...use of a systematic planning approach to develop acceptance or performance criteria for all work covered" and "assessment of existing data, when used to support Agency decisions or other secondary purposes, to verify that they are of sufficient quantity and adequate quality for their intended use."
Requirements for QA plans for data collection and modeling activities is one of the EPA's major means to achieve its high quality assurance goals.
Further Insight:
Guidelines for Ensuring and Maximizing the Quality, Objectivity, Utility, and Integrity of Information Disseminated by the Environmental Protection Agency (PDF) (66 pp, 896 K, About PDF) 2002. EPA-260R-02-008. Office of Environmental Information. U.S. Environmental Protection Agency. Washington, DC.
QAPP For Modeling
The EPA's Quality System for Environmental Data and Technology is in place to manage the quality of its environmental data collection, generation, and use (including models). Guidelines provide information about how to document and conduct quality assurance planning for modeling. Specific recommendations include:
- Specifications for developing assessment criteria
- Assessments at various stages of the modeling process
- Reports to management as feedback for corrective action
- The process for acceptance, rejection, or qualification of the output for the intended use
QAPP For Modeling (cont.)
A graded approach is also practical in the development of quality assurance project plan (QAPP) for modeling (EPA, 2002b). When models are developed or applied, the intended use of the generated results should be known. This information provides guidance for determining the appropriate level of quality assurance.
Data Quality Assessment
In some instances, the modeling project may utilize data coming from both direct and indirect measurements. A QA plan for data quality should identify:
- The need and intended use of each type of data or information to be acquired.
- Requirements on how indirect measurements are to be acquired and used
- How the data will be identified or acquired, and expected sources of these data.
- The method of determining the quality of the data.
- The criteria established for determining the quantity and quality level of data that is acceptable.
A Modeling Caveat
The EPA recommends using the terms 'precision' and 'bias,' rather than 'accuracy,' to convey the information usually associated with accuracy (the closeness of a measured or computed value to its 'true' value)
Acceptance Criteria for Individual Data Values (EPA, 2009a)
- Were the data collected from a population sufficiently similar to the population of interest within the context of model application?
- Would any characteristics of the dataset have an unintentional and direct impact the model output?
- In probabilistic models, are there adequate data in the upper and lower extremes of the tails to allow for unbiased probabilistic estimates?
- How is the uncertainty in the results estimated?
- Is the estimate of variability sufficiently small to meet the uncertainty objectives of the modeling project
Data Quality Assessment (cont.)
Acceptance Criteria for Individual Data Values (EPA, 2009a)
Qualifiers:
- Have the data met the quality assurances and data quality objectives?
- Is the system of qualifying or flagging data adequately documented?
Summarization:
- Is the data summarization process clear and sufficiently consistent with the goals of this project?
- Processing and transformation equations should be made available so that the underlying assumptions can be evaluated against the objectives of the project.
Peer Review
The peer review process provides the main mechanism for independent evaluation and review of environmental models used by the EPA. Its purpose is two-fold:
- To evaluate whether the assumptions, methods, and conclusions derived from environmental models are based on sound scientific principles
- To check the scientific appropriateness of a model for informing a specific regulatory decision
Peer review can uncover technical problems, oversights, or unresolved issues in preliminary versions of the model (EPA, 2006b).
Further Insight:
Peer Review Handbook. 2006. EPA/100/B-06/002. Science Policy Council, US Environmental Protection Agency Washington, DC.
Best Practices
The peer reviewpeer reviewIn EMAP, peer review means written, critical response provided by scientists and other technically qualified participants in the process. EMAP documents are subject to formal peer review procedures at laboratory and program levels. In EMAP, Level 1 peer reviews are performed by EPA's Science Advisory Board, level 2 by the NAS National Research Council, level 3 by specialist panel peer reviews, and level 4 by internal EPA respondents. process has an important role in each stage of the model lifecycle. Mechanisms for external peer review could include (EPA, 1994):
- Using an ad hoc panel of scientists
- Using an established peer review mechanism (e.g. Science Advisory Board, Committee on Environment and Natural Resources Research)
- Holding a technical workshop
The peer review process should also be well documented. In the earliest stages of model development, the team should identify the expected evaluation events and peer review processes. During any peer review process the review panel would also be presented with charge questions to drive the review.
Best Practices (cont.)
Peer Review Elements
Critical Elements of the Peer Review Process Individual Data Values (EPA, 1994; 2009a)
- Modeling Purpose/Objectives (context, application niche
application nicheThe set of conditions under which the use of a model is scientifically defensible. The identification of application niche is a key step during model development. Peer review should include an evaluation of application niche. An explicit statement of application niche helps decision makers to understand the limitations of the scientific basis of the model.)
- Major Considerations (processes, scales, etc.)
- Theoretical Basis of the Model (shortcomings, algorithms)
- Parameter
ParameterConstants applied to a model that are obtained by theoretical calculation or measurements taken at another time and/or place, and are assumed to be appropriate for the place and time being studied. Estimation (methods, boundary conditions)
- Data Quality/Quantity (data adequacy, selection process)
- Key Assumptions (basis of, sensitivity to)
- Model Performance Measures (criteria, relative performance)
- Model Documentation (comprehensive)
- Retrospective (were intentions realized, robustness, uncertainty quantification)
Application Niche: The set of conditions under which the use of a model is scientifically defensible. The identification of application niche is a key step during model development.
Example Charge Questions
Examples of charge questions for a peer review process are provided to the right. For additional information on these models please see the citations and links provided below.
Second Generation Model (SGM)
The Science Advisory Board Review of EPA's Second Generation Model (EPA, 2007a)
The Second Generation Model (SGM) is a computable general equilibrium model designed specifically to analyze issues related to energy, economy, and greenhouse gas emissions. These questions represent general and over arching questions charged to the peer review group. See EPA (2007a) for the full report and more specific questions.
- Is the SGM appropriate and useful for answering questions on the economic effects of climate policies?
- Are the model's structure and fundamental assumptions reasonable and consistent with economic theory?
- Are the parameter
parameterConstants applied to a model that are obtained by theoretical calculation or measurements taken at another time and/or place, and are assumed to be appropriate for the place and time being studied. values employed in the model (e.g. elasticities of substitution and of demand, price and income) within the range of values in the literature?
- Are the model's parameterizations logical?
- Are the model's projections of future energy use and efficiency reasonable, given fundamental physical constraints and rates of technological change?
- In what areas is the model in need of further development?
Example Charge Questions (cont.)
EPI Suite™ Model
The Science Advisory Board Review of the Estimation Programs Interface Suite (EPI Suite™) (EPA, 2007b)
The EPI Suite™ is suite of physical/chemical property and environmental fate estimation models developed by the EPA's Office of Pollution Prevention Toxics and Syracuse Research Corporation (SRC). These questions represent general and over arching questions charged to the peer review group. See EPA (2007b) for the full report and more specific questions.
- Are there additional properties that should be included in upgrades to the model for its various specified uses?
- Are there places where EPI Suite™'s user guide (and other program documentation) does not clearly explain EPI's design and use? How can these be improved?
- Are there aspects of the user interface that need to be corrected, redesigned, or otherwise improved?
- Are there other features that could enhance convenience and overall utility for users?
- Is adequate information on accuracy/validation conveyed to the user by the program documentation and/or the program itself?
Corroboration
Definitions
Assessing the degree to which a model represents a defined system is not simply a matter of comparing model results and empirical data. During the Development Stage, the modeling team determined an acceptable degree of total uncertaintyuncertaintyThe term used in this guidance to describe lack of knowledge about models, parameters, constants, data, and beliefs. There are many sources of uncertainty, including the science underlying a model, uncertainty in model parameters and input data, observation error, and code uncertainty. Additional study and collecting more information allows error that stems from uncertainty to be minimized/reduced (or eliminated). In contrast, variability (see definition) is irreducible but can be better characterized or represented with further study. within the context of specific model applications. Ideally, this determination should be informed by decision makers and stake holders; and described in a quality assurance plan.
Model corroboration assesses the degree to which a model corresponds to reality, using both quantitative and qualitative methods. The modelers may use a graded approach, as mentioned earlier, to determine the rigor of these assessments which should be appropriately defined for each model application.
Qualitative methods, like expert elicitation, can provide the development team with beliefs about a system's behavior in a data-poor situation. Utilizing the expert knowledge available, qualitative corroboration is achieved through consensus and consistency (EPA, 2009a).
Additional Web Resource:
Further information regarding the Development Stage can be found in the Best Modeling Practices: Development module.
Validation and Verification
Clarification on Model Evaluation, Validation and Verification
Model evaluation is defined as the process used to generate information to determine whether a model and its analytical results are of a quality sufficient to serve as the basis for a decision (EPA, 2009a).
Validated models are those that have been shown to successfully perform a specific task (model application scenario) of site-specific field data. Model validation is essentially problem specific since there are few 'universal' models (Beck et al., 1994). The Guidance Document (EPA, 2009a) focuses on the processes and techniques for model evaluation rather than model validation or invalidation. Please see the Case Study sub tab at the end of this section.
Verification is another term commonly applied to the evaluation process. However, model verification typically refers to model code. Verification is an assessment of the algorithms and numerical techniques used in the computer code to confirm that they work correctly and represent the conceptual model accurately - a process typically applied during the Development Stage (EPA, 2009a).
Further Insight:
Guidance on the Development, Evaluation, and Application of Environmental Models. 2009. EPA/100/K-09/003. Washington, DC. Office of the Science Advisor, US Environmental Protection Agency.
Models in Environmental Regulatory Decision Making. 2007. National Research Council. Washington, DC. National Academies Press.
Standard Guide for Statistical Evaluation of Atmospheric Dispersion Model Performance Exit (D 6589). ASTM. 2000.
Verification, Validation, and Confirmation of Numerical Models in the Earth Sciences. 1994. Oreskes, N., K. Shrader-Frechette and K. Belitz. Science 263 (5147): 641-646.
Quantitative Methods
Quantitative measures assess the robustness of a model - the capacity of a model to perform equally well across the full range of environmental conditions for which it was designed (EPA, 2009a). These assessments rely upon statistical measures to calculate various measures of fit between modeled results and measured data.
Model performance measures assess how close modeled results are to the measured data through deviances. Each method (Janssen and Heuberger, 1995) has strengths and weaknesses that should be considered when choosing an assessment measure. For example, modeling efficiency (ME) is a dimensionless statistic which directly relates model predictions to observed data and root mean square error (RMSE) is a method which is sensitive to outliers, but can accurately describe relationships between modeled data and noise-free (measured) data.
Graphical Methods
Simple plots between modeled and measured data can reveal qualitative assessments of model performance (i.e. time series, scatter plots, quantile-quantile (Q-Q) plots, spatial concentration plots, etc.).
Plotting modeled data against measured data is a simple way to assess model performance, as depicted in the figure to the right. The 1:1 line represents a model that is accurately predicting measured data.
Comparisons between Measured and Modeled Data along the '1:1 Line.' This simple comparison can be useful in early stages of model evaluation as a qualitative way to assess model performance.
Model Selection
In many scenarios, there may be a number of models suited for a particular application and the project team uses both quantitative and qualitative methods of model evaluation to select the best model for their modeling application.
Ranking models on the basis of their statistical performance against measured data can aid in the process of model selection. When quantitative measures of models performance do not distinguish one model from another, model selection can shift to a more qualitative nature. Past use, public familiarity, cost or resource requirements, and availability can all be useful metrics to help determine the most suitable model.
Additional Web Resource:
Further discussion of model selection can be found in the Best Modeling Practices: Application module.
Case Study: Validation
Application of AQUATOX v1.66 to Lake Onondaga, NY
- Registry of EPA Applications, Models and Databases (READ)
- AQUATOX home page
- Validation reports (EPA, 2000)
The aquatic ecosystem model AQUATOX is one of the few general ecological risk models that represents the combined environmental fate of various pollutants and their effects on the ecosystem, including fish, invertebrates, and aquatic plants (EPA, 2000).
In this example, AQUATOX was validated for use at Lake Onondaga, NY. The validation report (EPA, 2000) highlights multiple levels of validation with calibrated versions of the model.
Dissolved oxygen concentrations in the Lake Onondoaga hypolimnion in 1990. AQUATOX predictions indicate anoxic conditions in the middle of summer and the episode is remarkably close to the observed conditions (EPA, 2000).
Sensitivity Analysis (SA)
The purpose of a sensitivity analysis (SA) can be two-fold. First, SA computes the effect of changes in model inputs on the outputs. Second, SA can be used to study how uncertainty in a model output can be systematically apportioned to different sources of uncertainty in the model input.
Sensitivity analysis is defined as the computation of the effect of changes in input values or assumptions (including boundaries and model functional form) on the outputs. In other terms: how sensitive are the results to changes in the inputs, parameters, or model assumptions.
A non-intensive sensitivity analysis can first be applied to identify the most sensitive inputs. By discovering the 'relative sensitivity' of model parameters, the model development team is then aware of the relative importance of parameters in the model and can select a subset of the inputs for more rigorous sensitivity analyses (EPA, 2009a). This also ensures that a single parameter is not overly influencing the results.
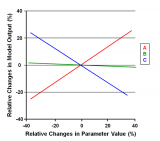
A spider diagram used to compare relative changes in model output to relative changes in the parameter values can reveal sensitivities for each parameter (Addiscott, 1993). In this example, the effects of changing parameters A, B, and C are compared to relative changes in model output. The legs represent the extent and direction of the effects of changing parameter values. (Click on image for a larger version)
Sensitivity Analysis Methods
There are many methods for sensitivity analysis (SA), a few of which were highlighted in the Guidance on the Development, Evaluation, and Application of Environmental Models (EPA, 2009a). The chosen method is dependent upon assumptions made and the amount of information needed from the analysis. Those methods are categorized into:
- Screening Tools
- Morris's One-at-a-Time
- Differential Analysis Methods
- Methods Based on Sampling
- Variance Based Methods
For many of the methods it is important to consider the geometry of the response plane and potential interactions among parameters and/or input variables. Depending on underlying assumptions of the model, it may be best to start SA with simple methods to initially identify the most sensitive inputs and then apply more intensive methods to those inputs.
Physiologically based pharmacokinetic (PBPK) models represent an important class of dosimetry models that are useful for predicting internal dose at target organs for risk assessment applications (EPA 2006a). This figure is an example of the Monte Carlo simulation method for Sensitivity Analysis. The distribution of internal concentration versus time (output) is simulated by repeatedly (often as many as 10,000 iterations) sampling input values based on the distributions of individual parameters (blood flow rate, body weight, metabolic enzymes, partition coefficients, etc.) in a population. Adapted from EPA (2006a).
Further Insight
Sensitivity analysis (SA) is an important component of Model Evaluation. When combined with uncertainty analysis (UA) the contribution of input parameters to total uncertainty can be revealed. Further, knowing which inputs to focus further analyses on saves the research team valuable time.
There are many methods for SA, each coming with a set of caveats and features that can be used to select the best SA for a specific model application.
Additional Web Resource:
Specific methodologies are explored in the Sensitivity and Uncertainty Analyses module.
Further Insight:
Guiding Principles for Monte Carlo Analysis. 1997. EPA-630-R-97-001. Risk Assessment Forum. U.S. Environmental Protection Agency. Washington, DC.
Multimedia, Multi pathway, and Multi receptor Risk Assessment (3MRA) Modeling System Volume IV: Evaluating Uncertainty and Sensitivity (PDF) (19 pp, 203 K, About PDF). 2003. EPA530-D-03-001d. Office of Research and Development. US Environmental Protection Agency. Athens, GA.
Guidance on the Development, Evaluation, and Application of Environmental Models. 2009. EPA/100/K-09/003. Washington, DC. Office of the Science Advisor, US Environmental Protection Agency.
Uncertainty Analysis
Uncertainties (i.e. a lack of knowledge) are present and inherent throughout the modeling process. However, models can continue to be valuable tools for informing decisions through proper quantification and communication of the associated uncertainties (EPA, 2009a).
Uncertainty analysis (UA) investigates the effects of lack of knowledge or potential errors on model output. When UA is conducted in combination with sensitivity analysis; the model user can become more informed about the confidence that can be placed in model results (EPA, 2009a).
Model Uncertainty (EPA, 2009a)
-
Application niche uncertainty – uncertainty attributed to the appropriateness of a model for use under a specific set of conditions (i.e. a model application scenario).
-
Structure/framework uncertainty – incomplete knowledge about factors that control the behavior of the system being modeled; limitations in spatial or temporal resolution; and simplifications of the system.
-
Input/data uncertainty – resulting from data measurement errors; inconsistencies between measured values and those used by the model; also includes parameter value uncertainty.
Uncertainty and Variability
Uncertainty represents lack of knowledge about something that is true. It is a general term that is often applied in a number of contexts. In environmental modeling, it may describe a lack of knowledge about models, parameters, constants, data, or the underlying assumptions.
The nature of uncertainty can be described as (Walker et al., 2003; Pascual 2005; EPA, 2009b):
- Stochastic uncertainty – resulting from errors in empirical measurements or from the world's inherent stochastically
- Epistemic uncertainty – uncertainty from imperfect knowledge
- Technical uncertainty – uncertainty associated with calculation errors, numerical approximations, and errors in the model algorithms
Variability vs. Uncertainty
Variability is a special instance of uncertainty - often called data uncertainty. Variability of environmental data is a product of the inherent randomness and heterogeneity of the environment.
Variability can be better characterized, but hard to reduce, with further study.
Separating variability and uncertainty is necessary to provide greater accountability and transparency (EPA, 1997).
Uncertainty Matrix
Many of the modeling uncertainties identified have limited room for improvement. However, there are methods to resolving model uncertainty; even some of the qualitative uncertainties can be addressed.
Uncertainty analysis begins with characterizing the associated modeling uncertainties; often accomplished using a framework or uncertainty matrix (i.e. Walker et al., 2003; Refsgaard et al., 2007). Walker et al. (2003) discuss uncertainty as a 3-Dimensional relationship:
Location: Where the uncertainty manifests itself within the model complex (application niche, framework, or input uncertainty).
Level: The degree of uncertainty along the spectrum between deterministic knowledge and total ignorance.
Nature: whether the uncertainty comes from epistemic uncertainty, or the inherent variability of the phenomena being described.
Uncertainty Analysis Priorities
Reducing application niche uncertainty should be a first priority during a modeling exercise (EPA, 2009a). The application nicheapplication nicheThe set of conditions under which the use of a model is scientifically defensible. The identification of application niche is a key step during model development. Peer review should include an evaluation of application niche. An explicit statement of application niche helps decision makers to understand the limitations of the scientific basis of the model. of a model should be used to determine whether the use of a given model is appropriate for the situation. Other priorities include:
- Mapping the model attributes to the problem statement
- Confirming the degree of certainty needed from model outputs
- Determining the amount of reliable data available or the resources available to collect more
- The quality of the scientific foundations of the model
- The technical competence of the model development / application team
Model Attributes:
The processes (chemical, biological, physical); variables; scale; and outputs described or contained within the model.
Further Insight
The EPA has produced a number of resources and guidance documents on uncertainty analysis that are specific to a variety of environmental modeling fields. A few of those resources are identified to the right.
Additional Web Resource:
These methods are discussed further in the Sensitivity and Uncertainty Analyses module.
Further Insight:
Uncertainty and Variability in Physiologically Based Pharmacokinetic Models: Key Issues and Case Studies (10 pp, 69K, About PDF) 2008. EPA/600/R-08/090 Office of Research and Development. US Environmental Protection Agency. Washington, DC.
Guidance on the Development, Evaluation, and Application of Environmental Models. 2009. EPA/100/K-09/003. Office of the Science Advisor. US Environmental Protection Agency Washington, DC.
Using Probabilistic Methods to Enhance the Role of Risk Analysis in Decision-Making With Case Study Examples DRAFT 2009. EPA/100/R-09/001. Risk Assessment Forum. US Environmental Protection Agency. Washington, DC.
Summary
The purpose of this module is to explore the topic of model evaluation and identify the 'best modeling practices' and strategies for the Evaluation Stage of the model life-cycle. In summary:
- Model evaluation is a process of many activities that should include:
- Peer review
- Quality Assurance (QA) project planning
- Model corroboration
- Sensitivity analysis
- Uncertainty analysis
- Model evaluation should be conducted using a graded approach that is adequate and appropriate to the objectives of the modeling exercise.
- The peer review process provides the main mechanism for independent evaluation and review of environmental models used by the EPA.
- QA project planning promotes model transparency.
- There are many techniques and approaches for model corroboration. An appropriate method should be determined at the beginning of the model life-cycle.
- When practiced together, sensitivity and uncertainty analyses can used to study how uncertainty in a model output can be systematically apportioned to different sources of uncertainty in the model input.
Additional Web Resources:
-
SuperMUSE Website: Ecosystems Research Division's Supercomputer for Model Uncertainty and Sensitivity Evaluation (SuperMUSE) is a key to enhancing quality assurance in environmental models and applications.
You Have Reached The End Of The Best Modeling Practices: Development Module.
From here you can:
- Continue exploring this module by navigating the tabs and sub tabs
- Return to the Training Module Homepage
- Continue on to another module:
- You can also access the Guidance Document on the Development, Evaluation and Application of Environmental Models, March, 2009.
References
- Addiscott, T. M. 1993. Simulation modelling and soil behavior. Geoderma 60(1-4): 15-40.
- Beck, B., L. Mulkey and T. Barnwell. 1994. Model Validation for Exposure Assessments. DRAFT. Athens, GA: US Environmental Protection Agency.
- Cullen, A. C. and H. C. Frey 1999. Probabilistic Techniques in Exposure Assessment: A Handbook for Dealing with Variability and Uncertainty in Models and Inputs. New York. Plenum Press
- EPA (U.S. Environmental Protection Agency). 1994. Report of the Agency Task Force on Environmental Regulatory Modeling. EPA 500-R-94-001. Solid Waste and Emergency Response.
- EPA (US Environmental Protection Agency). 2000. AQUATOX For Windows: A Modular Fate and Effects Model for Aquatic Ecosystems: Release 1 Volume 3: Model Validation Reports. EPA-823-R-00-008. Washington, DC. Office of Water
- EPA (U.S. Environmental Protection Agency). 2002a. Guidance on Environmental Data Verification and Data Validation EPA QA/G-8 (PDF) (96 pp, 386 K, About PDF). EPA/240/R-02/004. Washington, DC. Office of Environmental Information.
- EPA (U.S. Environmental Protection Agency). 2002b. Guidelines for Ensuring and Maximizing the Quality, Objectivity, Utility, and Integrity of Information Disseminated by the Environmental Protection Agency (PDF) (61 pp, 895 K, About PDF). EPA-260R-02-008. Washington, DC. Office of Environmental Information.
- EPA (US Environmental Protection Agency). 2003. Multimedia, Multi pathway, and Multi receptor Risk Assessment (3MRA) Modeling System Volume IV: Evaluating Uncertainty and Sensitivity (PDF) (19 pp, 203 K, About PDF). EPA530-D-03-001d. Athens, GA. Office of Research and Development.
- EPA (US Environmental Protection Agency). 2006a. Approaches for the Application of Physiologically Based Pharmacokinetic (PBPK) Models and Supporting Data in Risk Assessment (PDF) (10 pp, 68 K, About PDF). EPA/600/R-05/043F. Washington, DC. Office of Research and Development.
- EPA (US Environmental Protection Agency). 2006b. Peer Review Handbook EPA/100/B-06/002. Washington, DC. Science Policy Council.
References (Continued)
- EPA (US Environmental Protection Agency). 2007a. SAB Advisory on EPA's Second Generation Model (PDF) (46 pp, 403 K, About PDF). EPA-SAB-07-006. Washington, DC. Science Advisory Board
- EPA (US Environmental Protection Agency). 2007b. Science Advisory Board (SAB) Review of the Estimation Programs Interface Suite (EPI SuiteTM) (PDF) (60 pp, 473 K, About PDF). EPA-SAB-07-011. Washington, DC. Science Advisory Board
- EPA (US Environmental Protection Agency). 2009a. Guidance on the Development, Evaluation, and Application of Environmental Models EPA/100/K-09/003. Washington, DC. Office of the Science Advisor.
- EPA (US Environmental Protection Agency). 2009b. Using Probabilistic Methods to Enhance the Role of Risk Analysis in Decision-Making With Case Study Examples. DRAFT. EPA/100/R-09/001 Washington, DC. Risk Assessment Forum.
- Hanna, S. R. 1988. Air quality model evaluation and uncertainty. Journal of the Air Pollution Control Association 38(4): 406-412.
- Jakeman, A. J., R. A. Letcher and J. P. Norton 2006. Ten iterative steps in development and evaluation of environmental models. Environmental Modeling & Software 21(5): 602-614.
- Janssen, P. H. M. and P. S. C. Heuberger 1995. Calibration of process-oriented models. Ecol. Model. 83(1-2): 55-66.
- Matott, L. S., J. E. Babendreier and S. T. Purucker 2009. Evaluating uncertainty in integrated environmental models: A review of concepts and tools. Water Resour. Res. 45: Article Number: W06421.
- NRC (National Research Council) 2007. Models in Environmental Regulatory Decision Making. Washington, DC. National Academies Press.
- Pascual, P. 2005. Wresting Environmental Decisions From an Uncertain World. Environmental Law Reporter 35: 10539-10549
- Refsgaard, J. C., J. P. van der Sluijs, A. L. Højberg and P. A. Vanrolleghem 2007. Uncertainty in the environmental modeling process - A framework and guidance. Environ. Model. Software 22(11): 1543-1556.
- Saltelli, A., K. Chan, and M. Scott, eds. 2000. Sensitivity Analysis. New York: John Wiley and Sons.
- Sunderland, E. 2008. Addressing Model Uncertainty & Best Practices for Model Evaluation. Presentation at Presentation at Region 1 Regional Science Council Models Training Course. October 23, 2008.
- Walker, W. E., P. Harremoës, J. Rotmans, J. P. van der Sluijs, M. B. A. van Asselt, P. Janssen and M. P. Krayer von Krauss 2003. Defining Uncertainty: A Conceptual Basis for Uncertainty Management in Model-Based Decision Support. Integrated Assessment 4(1): 5-17.